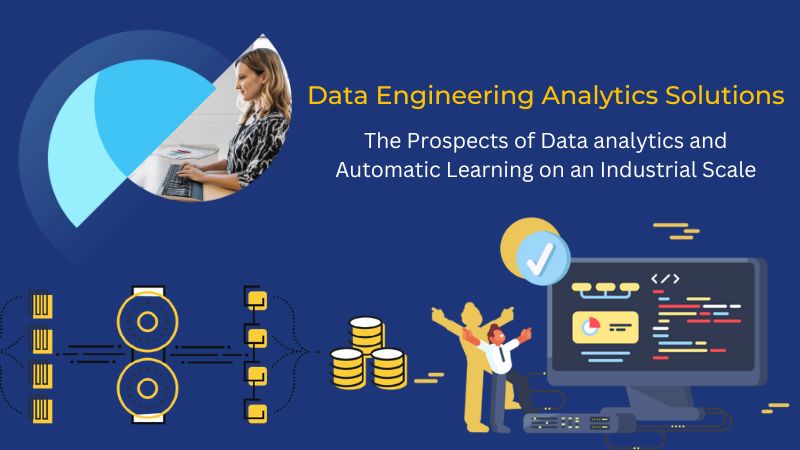
The Prospects of Data analytics and Automatic Learning on an Industrial Scale
You have most likely been familiar with the words “data science,” “artificial intelligence,” “analytics,” and “machine learning” when used to an enterprise setting.
The Question Remains, What Is Data Science?
The collection of data and its preparation for analysis is the overarching goal of the many subfields, technologies, skills, and bodies of knowledge that make up what is known as “data science.” Parallel to this, we need to quantify the data, determine where it came from, and organize it in order to make it usable with analytics. This can be accomplished by securing the data, cleaning it, aggregating it, and manipulating it so that it can be consumed by analytical routines, solvers, packages, models, data warehouses, and data archives. This accumulation of data is the primary goal of the field of Data Science.
Data scientists make use of a wide array of tools, algorithms, and equations, as well as other types of machine learning strategies, in order to discover hidden patterns concealed within unstructured data. After that, these patterns may be used to help guide decision-making and increase comprehension of a variety of areas. Data science goes beyond the traditional practice of just crunching numbers to uncover the “why” behind your data.
Data engineering is a rapidly developing field
Ten years ago, the function of a data engineer was almost nonexistent in most organizations. However, there has been an increase in demand for this specific kind of software developer. The function changed as the field became more developed over time.
The duties of a data engineer might differ greatly from one organization to the next, and the function does not advance at the same rate in every organization. However, we see changes in the job in the following three areas:
- Data engineers will make extensive use of cloud computing and software as a service (SaaS) offerings.
- Less time will be spent developing and more time will be spent monitoring by data engineers.
- In this transition, data engineers will move from the feature teams to the foundation teams.
The Application of Data Engineering to the Industrial Markets
When working in the financial markets, data engineering analytics solutions can be responsible for doing routine tasks such as collecting data and cleaning the data, which involves removing problems such as duplication from the data. In the last stage, the deal will be automated with the assistance of the cleansed data.
In addition to this, data engineering contributes to the improvement of the financial markets in a variety of other ways. These include:
Risk management
The management of risk is an incredibly crucial part of any financial organization, making the function of the data engineer a vital one in this industry. Errors in the forecast of trades may be avoided with the use of data sets that have been thoroughly cleaned. This is significant because if the machine learning model is given incorrect data to train on, it will result in the investor suffering ongoing financial losses.
Analytics predicative
The investor will be able to anticipate the data trends and will be able to take the appropriate steps for the same in the here and now with the assistance of predictive analytics. When investing in the stock market, having the assistance of a data engineer may ensure that the appropriate choices are made by the company, person, or other entity. For example, if the machine learning model is given data that contains duplicates or anomalies, this will result in the model providing incorrect input. This erroneous input, in turn, will make for inaccurate forecasts in the transaction, which will result in less profit for the trader.
Fraud detection
In the future, data engineering analytics solutions would also support the identification of fraudulent activity. It is very necessary to have the data examined and cleaned by a data engineer since it is of the utmost significance to determine whether or not a hacker has broken into the system with the intention of making the data harmful or unsuitable for feeding the predictive model.
Trading based on algorithms
In the realm of algorithmic trading in data engineers assist with the cleansing of the data that is to be provided to the machine learning or deep learning models in order to anticipate the trades. These models are used to analyze and predict the outcomes of transactions. The orders are carried out by an algorithmic trading system with the assistance of instructions that have been pre-programmed.
Bottom Line
Since datasets are the most critical factors to consider when making choices, data engineering analytics solutions play an essential part in the operation of any business or the trading industry. In addition, the future of data engineering looks promising because of the continued development of technology and the growing need for the application of large amounts of data.


Average Rating